The iPDM-GO project aimed to improve diabetes care by applying the “integrated Personalised Diabetes Management” (iPDM) approach in Denmark. The goal was to enhance the iPDM approach with innovative personalized health technology and implement outcomes-based healthcare payment. The ultimate goal was to encourage individualized treatment of diabetes, as well as deploy payment systems that reward better healthcare, throughout Europe.
Diabetes – especially Type 2 Diabetes (T2D) – is a huge and multidimensional challenge for European societies. The International Diabetes Federation estimates that approximately 540 million people live with diabetes worldwide with numbers continuing to rise. Consequently, the cost of diabetes care also continuously grows and becomes an increasingly global challenge. It is thus key to provide cost-effective health services that are tailored to each patient’s unique needs and requirements.
The “integrated Personalized Diabetes Management” (iPDM) approach offers a structured disease management process that leverages structured documentation and fosters communication between patients and their treating healthcare professionals (HCPs) [1]. The iPDM concept, consisting of six steps that are repeated over the continuum of care, has been shown to significantly improve glycemic outcomes for people living with T2D on insulin therapy in the randomized, controlled PDM-ProValue study program [2, 3, 4].
However, the original iPDM approach does not provide a digital patient-centric tool to facilitate the patient to be closer involved in the structured process; the patient is merely asked to collect blood glucose data using a standard Blood Glucose Monitor (BGM) in between visits to the clinic, while patient-reported data is collected on paper. The inclusion of additional digital patient-centric tools that augment the iPDM journey holds promise to further improve these outcomes for people living with T2D and was the aim of the iPDM-GO project [5].
In the iPDM-GO project, we (i.e., DTU) were responsible for the design and evaluation of such patient-centric tools, and came up with two:
- DiaFocus – a smartphone-based mHealth tool that supports the iPDM process by collecting behavioral and patient-reported healthcare information to improve communication between patients and their treating clinicians.
- EFT – a mHealth app that facilitates the creation and delivery of Episodic Future Thinking (EFT) as micro-interventions on a smartphone platform.
The DiaFocus System
The overall aim of the DiaFocus system is to support an adaptive assessment of the status of diabetes for a patient as part of the iPDM approach [1], as illustrated in Figure 1.
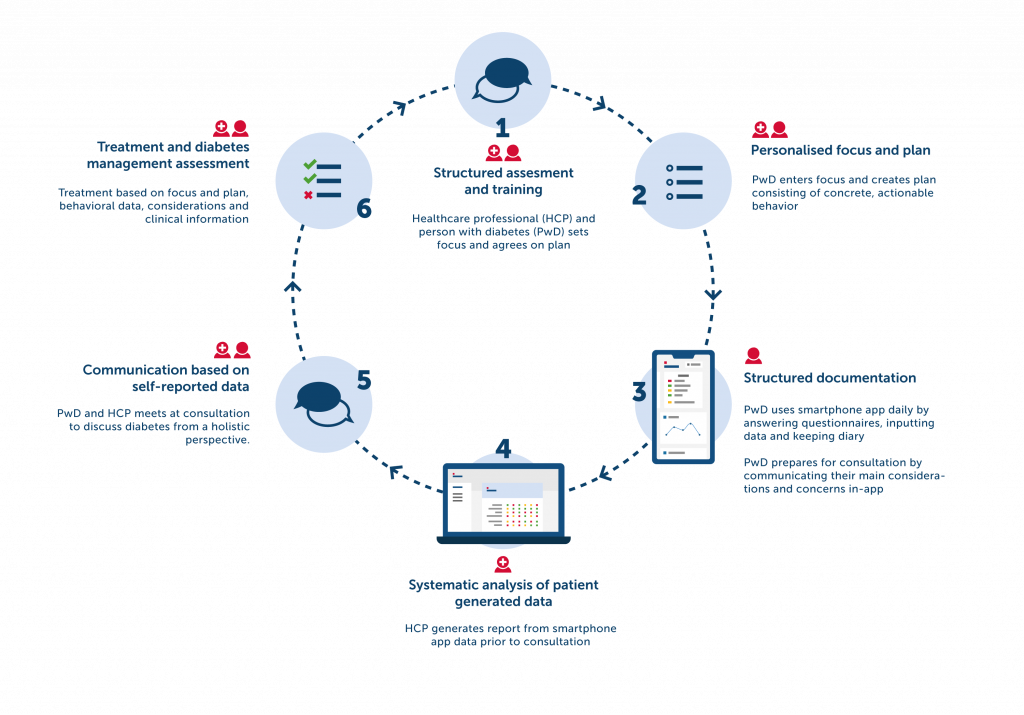
Figure 1 – The DiaFocus iPDM six-step cycle (from [6]).
DiaFocus is designed to be a patient-centric tool that keeps patients engaged in the iPDM treatment process. Specifically, DiaFocus implements a multi-sensory approach that combines passive mobile sensing of the patient’s behavior, physiological measures of blood glucose, experience sampling, and patient-reported healthcare information. DiaFocus implements an ‘adaptive assessment’ approach, which implies that the collection of sensor data and experience sampling is adapted according to the specific diabetes focus area, which is agreed upon between the patient and physician to improve and focus the patient-physician consultations. Moreover, in addition to the assessment of the behavioral and physiological aspects of the patient’s health, DiaFocus has a distinct focus on the assessment of the psycho-social aspects of handling diabetes over an extended period. This broad assessment and insight into the patient’s life support the patient-clinician communication and help structure the treatment and care in the iPDM treatment approach.
DiaFocus is implemented as an application in the Copenhagen Research Platform (CARP) and utilizes the Research Package for the collection of patient-reported data and the CARP Mobile Sensing Framework for the collection of sensor data on blood glucose, activity, and context. More detailed information on the design and technical architecture of DiaFocus is available in the 2023 ACM Health paper.
The EFT mHealth App
It has repeatedly been shown that a healthy lifestyle is key to preventing the transition from pre-diabetes to Type 2 Diabetes (T2D) and to the self-management of T2D. However, despite the transition being largely avoidable and medication can be significantly reduced through lifestyle changes and weight loss, the uptake in prevention programs remains low. This is mainly because the faraway consequences of smaller unhealthy behaviors seem less relevant at the moment. For example, the benefit of eating a cake on the sofa rather than going for a run in the rain is much preferred at the moment, even though the latter is much better for long-term T2D health.
The human tendency to discount the future can be assessed via the method of ‘delay discounting’, a behavioral economic trans-disease process measuring the extent to which people prefer smaller immediate rewards over larger delayed ones.
One intervention shown to be effective at reducing delay discounting is Episodic Future Thinking (EFT). In EFT a person mentally projects him/herself into the future through a personal vivid and sufficiently detailed episodic future event. This can be facilitated by first asking the person to imagine one or more attractive future events, for instance, “I am playing football with the grandchildren on a summer day”, and associate these with a created audio cue, that can then afterward be used to recall the future event.
As part of the iPDM-GO project, we investigated how EFT-based micro-interventions could be supported by a mHealth application, aiming to support micro-interventions both stand-alone and as part of a larger narrative [7]. The EFT-based micro-intervention approach is based on a theoretical engagement into the theory behind EFT combined with a thorough design process, involving a wide range of chronic patients engaged in focus groups, formative usability testing, and an initial feasibility study of the mHealth application.
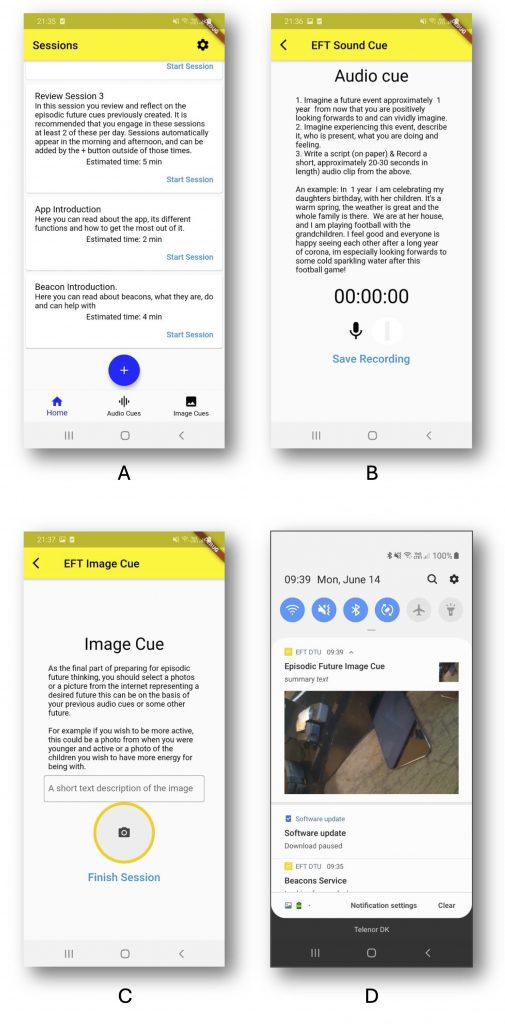
Figure 2 shows some of the main application screens of the EFT app. The EFT mobile health application was designed to facilitate the creation and delivery of EFT as micro-interventions on a smartphone platform. The smartphone presents a perfect platform for understanding the context and behavior of a person and for delivering an intervention through e.g., the notification system.
The EFT app supports three distinct micro-interventions (MIs) and one method for self-assessment (A):
- MI1 – A self-guided generation task for creating cues.
- MI2 – Audio-based projection (or review) session where audio cues are experienced.
- MI3 – Image-based projection micro-intervention where the image represents one of the episodic futures.
- A1 – An adjusting amounts task to assess delay discounting.
The EFT application was implemented in Flutter and is thus available for both Android and iOS smartphones. The software architecture is based on the Copenhagen Research Platform (CARP). The application leverages the CARP Mobile Sensing framework for mobile data collection, the CARP Research Package for collecting informed consent from the user, and the CARP Web Services infrastructure for secure data upload and management. The real-time collection of contextual and behavioral data is especially relevant for this EFT application, as it can be used for just-in-time or ecologically relevant triggers in the app. All data collected on the participants’ smartphones is sent to the CARP Web Service backend, where it is made accessible to the project researchers.
In addition to handling data collection, CARP Mobile Sensing also controls the timing of new sessions, scheduling of notifications, and delivery of image reminders through the Study Protocol.
The proposed approach was subsequently evaluated in a randomized feasibility study investigating the use of mHealth technology for EFT to improve delay discounting in health [8]. The study enrolled 208 participants over 21 days, who were assigned to either using the EFT app or a control group. The study investigated whether EFT micro-interventions can be delivered through mHealth technology to reduce delay discounting.
Results indicated that the intervention group achieved a significant reduction of delay discounting compared to the control group. Hence, the results indicate that EFT can work as micro-interventions and can modify discounting rates.
The study also investigated different types of EFT (“normal” EFT versus “goal-oriented” EFT) and did detailed studies of the perceived usefulness and usability of the EFT app. The 2024 Digital Health paper[8] contains much more details.
Funding
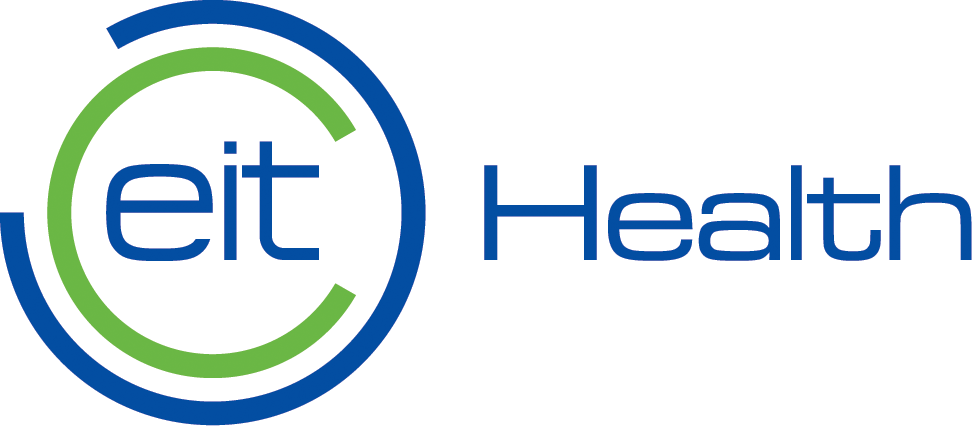
iPDM-GO was funded by EIT Health as an Innovation Project. EIT Health is supported by the European Institute of Innovation and Technology (EIT), a body of the European Union that receives support from the European Union’s Horizon Europe Research and Innovation Programme.
Partners
- Roche Diabetes Care (Project Coordinator)
- Technical University of Denmark, Department of Health Technology
- Steno Diabetes Center Copenhagen
- German Center for Diabetes Research
- Center for Diabetes, City of Copenhagen
- University of Copenhagen
- Helmholtz Forschungszentrum für Gesundheit und Umwelt
References
[Bibtex]
@article{ceriello2012diabetes,
title={Diabetes as a case study of chronic disease management with a personalized approach: the role of a structured feedback loop},
author={Ceriello, Antonio and Barkai, L{\'a}szl{\'o} and Christiansen, Jens Sandahl and Czupryniak, Leszek and Gomis, Ramon and Harno, Kari and Kulzer, Bernhard and Ludvigsson, Johnny and N{\'e}methyov{\'a}, Zuzana and Owens, David and others},
journal={Diabetes research and clinical practice},
volume={98},
number={1},
pages={5--10},
year={2012},
publisher={Elsevier}
}
[Bibtex]
@article{heinemann2020benefit,
title={Benefit of digital tools used for integrated personalized diabetes management: results from the PDM-ProValue study program},
author={Heinemann, Lutz and Schramm, Wendelin and Koenig, Helena and Moritz, Annette and Vesper, Iris and Weissmann, Joerg and Kulzer, Bernhard},
journal={Journal of diabetes science and technology},
volume={14},
number={2},
pages={240--249},
year={2020},
publisher={SAGE Publications Sage CA: Los Angeles, CA}
}
[Bibtex]
@article{kulzer2018integrated,
title={Integrated personalized diabetes management improves glycemic control in patients with insulin-treated type 2 diabetes: results of the PDM-ProValue study program},
author={Kulzer, Bernhard and Daenschel, Wilfried and Daenschel, Ingrid and Schramm, Wendelin and Messinger, Diethelm and Weissmann, Joerg and Vesper, Iris and Parkin, Christopher G and Heinemann, Lutz},
journal={diabetes research and clinical practice},
volume={144},
pages={200--212},
year={2018},
publisher={Elsevier}
}
[Bibtex]
@article{kulzer2016integrated,
title={Integrated Personalized Diabetes Management (PDM) Design of the ProValue Studies: Prospective, Cluster-Randomized, Controlled, Intervention Trials for Evaluation of the Effectiveness and Benefit of PDM in Patients With Insulin-Treated Type 2 Diabetes},
author={Kulzer, Bernhard and Daenschel, Wilfried and Daenschel, Ingrid and Siegel, Erhard G and Schramm, Wendelin and Parkin, Christopher G and Messinger, Diethelm and Weissmann, Joerg and Djuric, Zdenka and Mueller, Angelika and others},
journal={Journal of diabetes science and technology},
volume={10},
number={3},
pages={772--781},
year={2016},
publisher={SAGE Publications Sage CA: Los Angeles, CA}
}
![[pdf]](https://www.bardram.net/wp-content/plugins/papercite/img/pdf.png)
[Bibtex]
@article{jones2020,
author = {Jones, Allan and Bardram, Jakob Eyvind and B{\ae}kgaard, Per and Cramer-Petersen, Claus Lundgaard and Skinner, Timothy and Vrangb{\ae}k, Karsten and Starr, Laila and N{\o}rgaard, Kirsten and Lind, Nanna and Bechmann Christensen, Merete and Gl{\"{u}}mer, Charlotte and Wang-Sattler, Rui and Laxy, Michael and Brander, Erik and Heinemann, Lutz and Heise, Tim and Schliess, Freimut and Ladewig, Katharina and Kownatka, Dagmar},
issn = {1751-9918},
journal = {Primary Care Diabetes},
keywords = {Artificial intelligence,Delivery of health care,Diabetes mellitus,Patient reported outcome measures,Value-based health insurance,integrated,type 2},
title = {{Integrated personalized diabetes management goes Europe: A multi-disciplinary approach to innovating type 2 diabetes care in Europe}},
year = {2020}
}
![[pdf]](https://www.bardram.net/wp-content/plugins/papercite/img/pdf.png)
[Bibtex]
@article{bardram2023diafocus,
title={DiaFocus: a personal health technology for adaptive assessment in long-term management of Type 2 diabetes},
author={Bardram, Jakob E and Cramer-Petersen, Claus and Maxhuni, Alban and Christensen, Mads VS and B{\ae}kgaard, Per and Persson, Dan R and Lind, Nanna and Christensen, Merete B and N{\o}rgaard, Kirsten and Khakurel, Jayden and others},
journal={ACM Transactions on Computing for Healthcare},
volume={4},
number={2},
pages={1--43},
year={2023},
publisher={ACM New York, NY}
}
![[pdf]](https://www.bardram.net/wp-content/plugins/papercite/img/pdf.png)
[Bibtex]
@inproceedings{persson2023episodic,
title={Episodic future thinking as digital micro-interventions},
author={Persson, Dan Roland and Yoo, Soojeong and Bardram, Jakob E and Skinner, Timothy C and B{\ae}kgaard, Per},
booktitle={International Conference on Human-Computer Interaction},
pages={213--237},
year={2023},
organization={Springer Nature Switzerland Cham}
}
![[pdf]](https://www.bardram.net/wp-content/plugins/papercite/img/pdf.png)
[Bibtex]
@article{persson2024perceptions,
title={Perceptions and effectiveness of episodic future thinking as digital micro-interventions based on mobile health technology},
author={Persson, Dan Roland and Bardram, Jakob E and B{\ae}kgaard, Per},
journal={Digital Health},
volume={10},
pages={20552076241245583},
year={2024},
publisher={SAGE Publications Sage UK: London, England}
}